Untangling the web of model behaviours
Posted on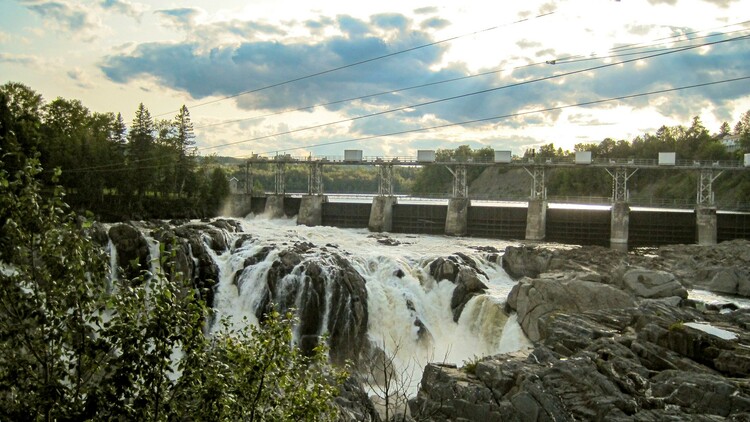
Grand Falls dam on the Saint John River in northern New Brunswick. Hydropower is one of the many variables the Davies lab is modelling using GCAM - Global Change Assessment Model. (Photo: Evan Arbuckle)
Understanding the future of energy systems brings together technology, environment, policy, and society - and it’s only getting more complex
It’s not predicting the future, it’s projecting.
That’s the vision behind the research being done in the group of Future Energy Systems researcher Evan Davies. Using complex computational models and reams of historical data, he is demonstrating what outcomes our national and global climate and energy choices can lead to. It’s a huge effort, and one that can only be tackled with the help of teams at the Joint Global Change Research Institute (JGCRI), Environment and Climate Change Canada (ECCC), and his team of graduate students, Diego Chiappori, Osama Younis, and Evan Arbuckle.
“Quite often, by the time you see a problem, it’s already huge,” Davies, an Associate Professor of Water Resources Engineering in the Department of Civil and Environmental Engineering, explains. “And too often, we think linearly.”
His work is part of an effort to defy these limitations, by opening up the entire system that surrounds energy to critical assessment and examination with the power of modern computing and systems analysis. The tool: the Global Change Analysis Model, or GCAM.
Started in the 1980s by JGCRI and under continuous improvement since, GCAM is an integrated assessment model that models our global society using real-life data, systems, and connections. It relies on deep collaboration and cooperation between researchers in many disciplines: engineering, economics, science, and policy to deliver a realistic, grounded view of how the world might look in 80 or 100 years, based on the energy and environment choices we make today.
Modelling global change
Originally, GCAM was begun as a proprietary model, emerging from the work of Jae Edmonds and John Reilly. However, in the last decade, GCAM modelling projects have begun in teams from around the world, with a mindset geared towards collaboration, not competition.
To accomplish their work, the Davies lab works closely with the researchers at JGCRI at the University of Maryland and the Economic Analysis Directorate at Environment and Climate Change Canada.
While the background operations of GCAM are necessarily complex, the main idea is straightforward. By breaking the world into 32 distinct geographical regions and relying on accurate and thorough historical data from across various aspects of society, the model projects how our interconnected global systems - energy, socioeconomics, land, water, climate, and emissions - will respond to change, and what those changes might look like. The types of change that can be simulated are virtually endless, ranging from the impacts of new policies to shifts in technology, the economy, or the energy sector.
“It’s like a sensitivity analysis – if we take a certain path, would we expect a lot of change or very little change,” Davies elaborates. “We can see feedback or knock-on effects, or we can see if solving one problem creates another. For example, does use of more renewables, like concentrating solar power, lead to more water use?”
The scope of the model is huge, and ever-increasing. Diego Chiappori has been involved since 2017 when he joined the Davies group as a PhD student fresh off a Masters modelling water resources in his home country of Brazil.
“It only works to look at it in the context of a system and that can’t be done by only one team,” he says. “No one understands the whole thing, we all add parts, what we’re specialized in.”
Evan Davies leads the GCAM research group at the University of Alberta.
GCAM isn’t designed for tracking small fluctuations. Like the difference between weather and climate, GCAM eschews day-to-day differences, running instead on an averaged data set of information collected over time.
“It simulates changes in areas in the world on a five year cycle, aimed to show long-term changes,” Davies explains.
That means that every five years, a new base year is calibrated, to serve as the jumping off point for any new projections.
In terms of energy, GCAM simulates both energy systems production in terms of resources used and electricity produced, as well as the demand for energy, but directly modelling energy is far from the only variable set. Also included are systems like buildings, regional and international markets, industry, cement production, fertilizer production, agriculture, land use, the global hydrological cycle including 235 different river basins, greenhouse gas emissions, climate change, and the effects of different climate policies.
“We look at how these are affected by climate change and different uses,” Diego explains. “Things like socio-economic change and economic performance – those factors are inputted, not modelled. [They are model drivers] that inform the model parameters.”
A Canadian spin on a global perspective
Peace Canyon Dam on the Peace River in northern British Columbia, immediately downstream of another, much larger hydropower installation, the W.A.C. Bennett Dam. (Photo: Evan Arbuckle)
An undertaking like GCAM development requires collaboration because the model incorporates and assesses a huge array of sectors and fields. The need to refine the model for each and every one of the 32 distinct global regions adds another layer of complexity.
Canada is currently represented as a single region in the GCAM model, but as the second largest country in the world with varied provincial landscapes, economies, and energy sectors, more resolution is needed. The Davies group has taken on the challenge of building a better model of Canada for GCAM, breaking the national average approach down into thirteen subregions –– one for each province and territory.
“We've had a lot of help and technical support from JGCRI, and lots of support and advice from ECCC as well,” Davies says. “It's been a team effort, and we really could not have done the work without them.”
The collaborative team include the Davies group at the University of Alberta, with Diego and fellow PhD student Osama Younis leading the project, two researchers at JGCRI, Matthew Binsted and Candelaria Bergero, and two members of ECCC, Nick Macaluso and Muhmmad-Shahid Siddiqui.
Diego began by assessing Canada’s socio-economics sector, such as the initial population and projected population growth including immigration, as well as the gross domestic product (GDP) for each province. He also had to account for data in the residential sector, aggregated industry, as well as the transportation sector. His work is nearing completion.
“The electrical sector is the last piece for me,” Diego says. “The model should be up and running in May.”
While his work over the past four years has incorporated untold gigabytes of data into the model, it’s only a first step. He offers an example: “Right now, only the cement and fertilizer industries are represented independently, due to their high greenhouse gas emissions. Osama will be responsible for further developing other areas.”
Developing those areas will bring GCAM’s model of Canada up to a level of detail only achieved for two other regions so far. First was the United States, which has already been broken down into its 51 states and territory. This GCAM-USA effort, with the involvement of Matthew Binsted, now collaborating with the Davies lab, has allowed the JGCRI team to better model the effects of specific regional policies, behaviours, and resources on the country, and the world. Another team has tackled diversification of the China geographical region, and more new regional models are incoming, including India, Saudi Arabia, Latin America, and others. Greater detail on a global scale improves the model’s simulation capabilities, making it a more useful tool.
Diego explains the significance: “When we run the model, we have to run all the regions. All these areas are connected – changing policies or technologies in one region affects the others, so they all have to be accurate.”
Fortunately, efforts to build more detail into different countries are collaborative, instead of competitive. “They help us check our assumptions, computer code, and data, to make sure we are being accurate,” Diego says. “It started at JGCRI, and we’re very grateful and lucky to have them involved in our own work. GCAM now is global, so it’s natural that we work together to refine it.”
Breaking it down
Diego Chiappori is working to split the GCAM-Canada model into thirteen separate sub-regions.
In general, energy-economy models such as GCAM can be categorized broadly by how they’re built: top-down or bottom-up. Top-down models focus on the big picture and the long term, representing technologies fairly generally. This can help in understanding general trade-offs to be had in changes, at the cost of dropping specific technical details. Bottom-up models are very technically accurate, at the cost of missing some of the bigger picture aspects that drive changes in complex systems. They also suffer in the realm of long-term projections, due to the inherent difficulty of predicting the changing technology of the future.
“Originally, the purpose of this model was top-down. Bigger scale, the overall picture,” Diego explains. “It was meant to ask: if one country does this, what effect does it have in other countries?” By accessing this tool, researchers could relate one sector in one country with an entirely different sector in another country.
“The problem is we always want more details and more information,” he continues. “Every time we add something to the model, it’s cool because we have more power to simulate. But if everyone’s adding everything, the model slows down tremendously.”
This raises an interesting point for the ongoing development of GCAM: as more detail is added, the lines between top-down and bottom-up modelling begin to blend. This can have significant impacts on the use of the model as a tool.
For example, hydrological studies – water, its uses and the natural cycle – was a fairly recent addition to the larger GCAM model. While understanding water impacts is critical to the environment, society and industry, adding hydro to the US model slowed computation by about twenty percent.
Niagara Falls is a valuable source of hydroelectric power generation.
“Running a single simulation can take an hour on a computer built for these purposes, or two hours on a normal computer. And sometimes you need to run 1000 simulations or scenarios to test different possibilities,” he points out.
Water is an area of particular interest to the Davies group: Masters student Evan Arbuckle recently published an analysis of true hydropower project costs, using GCAM to project the market share of hydropower in the energy system of the future.
Davies has also been co-author of other recent work from the JGCRI. One, published in Nature Communications, involved a collaborative assessment of a previously unaccounted for metric of virtual water trades – the water used for growth of exported agricultural products - from the present day to 2100. Work on the relative contributions of climate and socioeconomic systems on water scarcity was also recently published in Environmental Research.
“We often think that water resources will be hugely impacted by climate change, leading to scarcity,” Davies explains. “But we found that over the next 80 years, human demands – not climate change – are the bigger drivers of changes in water resources. That should have major implications on our behaviours.”
Seeing the water
Part of the Peace River valley that will be flooded within a few years with the completion of construction on the Site C dam, burying this farmland and habitat underwater but providing 1100 MW of electric generating capacity. (Photo: Evan Arbuckle)
The Davies group’s interest in water resources was one of the things that attracted Osama to the University of Alberta, after he completed his Masters in Environmental Management at the State University of New York.
“Water is a core module in the latest version of GCAM, demand and supply. There’s room for improvement though, and we are exploring better ways to do that,” he explains. “Specifically for municipal and industrial sectors – both account for about twenty percent of water demands in Canada.”
Canada is generally considered to be a water-secure country, but there are regions within the country that would greatly benefit from better management and better understanding of its interconnected supply and demand. Water demands in GCAM are based on other systems in the model, but the frameworks and connections to other systems are not completely developed. Improving the model’s ability to account for these feedbacks will require the collection of new data, and the integration of new linkages.
“How we model is based on our understanding of how a system or process works and this involves a lot of assumptions – which are based on a theoretical basis or an expert assessment,” Osama explains. “The model behaviour is as good as our assumptions, so we need to gather data to calibrate the model and validate its behaviour.”
One critical aspect of his assessment is understanding whether the water is withdrawn – returning back to the system after use – or consumed – meaning the water temporarily leaves the system.
“Water demands are a mix of withdrawal and consumption, and the ratio depends on the specific use,” he adds. Currently, thermal electric power generation is the largest water use in Canada, and is mostly a withdrawal scenario. On the other hand, a higher percentage of water withdrawn for agriculture, mining and oil and gas extraction is consumed.
Osama is currently focused on refining the industrial aspects of GCAM-Canada, so that the three sectors in Canada with the greatest water impact –– pulp and paper, primary metal manufacturing, and the chemicals manufacturing industries –– can be individually assessed.
“We’re trying to break down the black box into smaller compartments, and figure out how much water demand is allocated to each sector,” he explains. The ultimate goal is to include different sectors in GCAM so that the model can simulate scenarios that account for conflicting demands, and explore the potential impacts of regulation and technical changes in different sectors.
Connecting the systems
Diego Chiappori and Evan Arbuckle (second and third from left, respectively) present their research at the Research to the Real World Industry Mixer.
The sheer scale of the GCAM model might make any work to enhance it seem intimidating, but the members of the Davies group are motivated by the power the tool can provide decision makers.
“One of the great things about this field is that we can do analysis work that’s more open than usual,” Davies says. “We can learn what happens in this scenario versus what happens in another scenario – it’s unique.”
Diego concurs: “You can ‘create a policy’ in the model. For example, you could ask ‘what if in three years there were no fossil fuel cars on the road?’ and the model spits out everything, like how much more electricity China will need to produce to meet the demand from electric cars.”
“Focusing in the Candian context, there’s a big impact of the oil and gas sector, specifically unconventional oil, so we can see what the future of the oilsands industry might be,” Osama says.
He adds that an open mind is key, because results can be surprising when one system unexpectedly affects others. Being able to foresee unintended consequences and trade-offs is part of the motivation for working with GCAM – and one of the tool’s most useful features.
“We’re engineers, so we’re particularly interested in seeing the effects of technology change,” Davies says. “But it’s too simple to add a new technology and assume that it doesn’t have consequences for people, or the environment, or something else that we wouldn’t have thought about – until the model makes us ask the question.”
But in the end, Davies doesn’t believe assessments of technologies or policies are GCAM’s greatest power. Instead, he believes this sort of modelling can help people identify behaviours that can help, or see the value if behaviours change.
“What can we do to be better? Use less, be less wasteful as a society,” he says. “Demand better from manufacturers – things should be less disposable. But also find joy in things other than what’s the newest thing on the market.”
The GCAM modelling in the Davies lab clearly demonstrates that our societal, environmental, and energy systems are all deeply intertwined. Changes to policy, regulation, and agreed-upon norms will be necessary to alter our current climate and environmental trajectory – but modelling shows that it is possible. With COVID stay-at-home orders, for example, greenhouse gas emissions reduced dramatically, which Davies hopes will give people hope for climate action.
“It’s easy to stay focused on the four walls around us,” Davies concludes, “but in the future, other factors will make the decisions for us if we don’t make them ourselves.”
To learn more about the GCAM research ongoing in the Davies lab, click here.
You can also watch Evan Davies explain his work on the FES YouTube Channel.